I will be an area chair, ICCV 2021 I will be an area chair, CVPR 2021 1 May 2021: I am looking for a passionate and creative postdoc researcher to join the VisionCAIR group @KAUST (https://cemse.kaust.edu.sa/vision-cair). Key requirements include good communication (verbal, writing, etc) and excellent publication record in high-quality journals and/or conference proceedings such as CVPR, ICCV, NeurIPS, ICML, ICLR, ECCV, Nature, TPAMI, AAAI. It is an opportunity to make core algorithmic advances and aim at publishing them at these top venues. More details about the position can be found here. https://www.facebook.com/cemseKAUST/posts/2430594450578352. I have 1-2 grad student positions as well.
2021 | ArtEmis: Affective Language for Art Panos Achlioptas, Maks Ovsjanikov, Kilichbek Haydarov, Mohamed Elhoseiny, Leonidas Guibas, CVPR, 2021. A partnership project proposed while visiting Stanford 2019-2020, would not have been possible without the hard work by everyone and the excellent execution by Panos and Kilich.
My talk on "Imagination supervised Machines that can See, Create, Drive, and Feel" https://webcast.kaust.edu.sa/Mediasite/Showcase/default/Presentation/8e74a8cf69384ca3bbf4b88bc2addea51d
| paper link website https://www.artemisdataset.org/ | | Adversarial Generation of Continuous Images, Ivan Skorokhodo, Savva Ignatyev, Mohamed Elhoseiny, CVPR, 2021 | https://github.com/universome/inr-gan | | VisualGPT: Data-efficient Image Captioning by Balancing Visual Input and Linguistic Knowledge from Pretraining, Jun Chen, Han Guo, Kai Yi, Boyang Li, Mohamed Elhoseiny, Arxiv, 2021
| paper link | | Class Normalization for (Continual)? Zero-Shot Learning, Ivan Skorokhodov, Mohamed Elhoseiny, ICLR, 2021 | | | HalentNet: Multimodal Trajectory Forecasting with Hallucinative Intents, Deyao Zhu, Mohamed Zahran, Li Erran Li, Mohamed Elhoseiny, ICLR, 2021 | | | Semi-Supervised Few-Shot Learning with Prototypical Random Walks, Ahmed Ayyad, Yuchen Li, Raden Muaz, Shadi Albarqouni*, Mohamed Elhoseiny* long paper, MetaLern Workshop, AAAI (oral), 2021 https://metalearning.chalearn.org/ | | | | | 2020 | Temporal Positive-unlabeled Learning for Biomedical Hypothesis Generation via Risk Estimation. Uchenna Akujuobi Jun Chen, Mohamed Elhoseiny, Michael Spranger, Xiangliang Zhang, NeurIPS, 2020 | | | Panos Achlioptas, Ahmed Abdelreheem, Fei Xia, Mohamed Elhoseiny, Leonidas Guibas, ReferIt3D: Neural Listeners for Fine-Grained Object Identification in Real-World 3D Scenes [Oral], European Conference on Computer Vision (ECCV), 2020, | http://referit3d.github.io/ | | - Abduallah Mohamed, Kun Qian, Mohamed Elhoseiny**, Christian Claudel**
Social-STGCNN: A Social Spatio-Temporal Graph Convolutional Neural Network for Human Trajectory Prediction, CVPR, 2020, **co-advisors | https://github.com/abduallahmohamed/Social-STGCNN
| | Yuanpeng Li, Liang Zhao, Ken Church, Mohamed Elhoseiny. Compositional Continual Language Learning, ICLR, 2020 | [paper] | | Sayna Ebrahimi, Mohamed Elhoseiny, Trevor Darrell, Marcus Rohrbach Uncertainty-guided Continual Learning with Bayesian Neural Networks, ICLR, 2020
| [paper] | 2019 |
| | | Lia Coleman, Panos Achlioptas, Mohamed Elhoseiny Towards a Principled Evaluation of Machine Generated Art, NeurIPS creativity workshop, 2019 | [paper] [poster],
Featured in NeurIPS AI Art Gallery http://www.aiartonline.com/paper-demos-2019/lia-coleman-panos-achiloptas-mohamed-elhoseiny/
| | Mohamed Elhoseiny, Mohamed Elfeki Creativity Inspired Zero Shot Learning, Thirty-sixth International Conference on Computer Vision (ICCV), 2019 | [code] | | Mohamed Elfeki, Camille Couprie, Morgane Riviere, Mohamed Elhoseiny GDPP: Learning Diverse Generations Using Determinantal Point Processes Thirty-sixth International Conference on Machine Learning (ICML), 2019 | [code] | | Arslan Chaudhry, Marc’Aurelio Ranzato, Marcus Rohrbach, Mohamed Elhoseiny, Efficient Lifelong Learning with A-GEM, ICLR, 2019 | . [code] | | Ji Zhang,Yannis Khaladis, Marcus Rohbrach, Manohar Paluri, Ahmed Elgammal, Mohamed Elhoseiny, Large-Scale Visual Relationship Understanding, AAAI, 2019 | [code] | | Mennatullah Siam, Chen Jiang, Steven Lu, Laura Petrich, Mahmoud Gamal, Mohamed Elhoseiny, Martin Jagersand. Video Segmentation using Teacher-Student Adaptation in a Human Robot Interaction (HRI) Setting." ICRA, 2019 | [code] | 2018 | | | | Ramprasaath Selvaraju, Prithvijit Chattopadhyay, Mohamed Elhoseiny, Tilak Sharma, Dhruv Batra, Devi Parikh, Stefan Lee, Choose your Neuron: Incorporating Domain Knowledge through Neuron Importance, ECCV, 2018 | | | Mohamed Elhoseiny,Francesca Babiloni, Rahaf Aljundi, Manohar Paluri, Marcus Rohrbach, Tinne Tuytelaars, Exploring the Challenges towards Lifelong Fact Learning, ACCV 2018 https://arxiv.org/abs/1711.09601 | | | Rahaf Aljundi, Francesca Babiloni, Mohamed Elhoseiny, Marcus Rohrbach, Tinne Tuytelaars, Memory Aware Synapses: Learning what (not) to forget, ECCV 2018 https://arxiv.org/abs/1711.09601
| | | Othman Sbai, Mohamed Elhoseiny, Antoine Bordes, Yann LeCun, Camille Couprie, “DesIGN, Design Inspiration from Generative Networks”, ECCVW, September, 2018 https://arxiv.org/abs/1804.00921 Best Paper Award in the workshop | | | Imagine it for me: Generative Adversarial Approach for Zero-Shot Learning from Noisy Texts, CVPR, 2018 https://arxiv.org/abs/1712.01381
| Language&Vision | | Ahmed Elgammal, Bingchen Liu, Diana Kim, Mohamed Elhoseiny, Marian Mazzone, "The Shape of Art History in the Eyes of the Machine", AAAI, 2018 (to appear) | Art &Vision | | Mohamed Elhoseiny, Manohar Paluri, Yitzhe-Ethan Zhu, Ahmed Elgammal, "Language Guided Visual Recognition", Deep Learning Semantic Recognition Book, 2018 (to appear) | Book Chapter Language & Vision Area | 2017 | | | | Ahmed Elgammal, Bingchen Liu, Mohamed Elhoseiny and Marian Mazzone CAN: Creative Adversarial Networks, International Conference on Computational Creativity(ICCC), 2017 | Conference paper Art &Vision | | M Elhoseiny, A Elgammal, "Overlapping Cover Local Regression Machines", Arxiv, 2017 | pdf | | Mohamed Elhoseiny*, Yizhe Zhu*, Han Zhang, Ahmed Elgammal, Link the head to the "peak'': Zero Shot Learning from Noisy Text descriptions at Part Precision, CVPR, 2017 | Conference paper (To appear) Language & Vision
code will be available soon | | Ji Zhang*, Mohamed Elhoseiny*, Walter Chang, Scott Cohen, Ahmed Elgammal, "Relationship Proposal Networks", CVPR, 2017 | Conference paper (To appear) Pairwise region proposals | 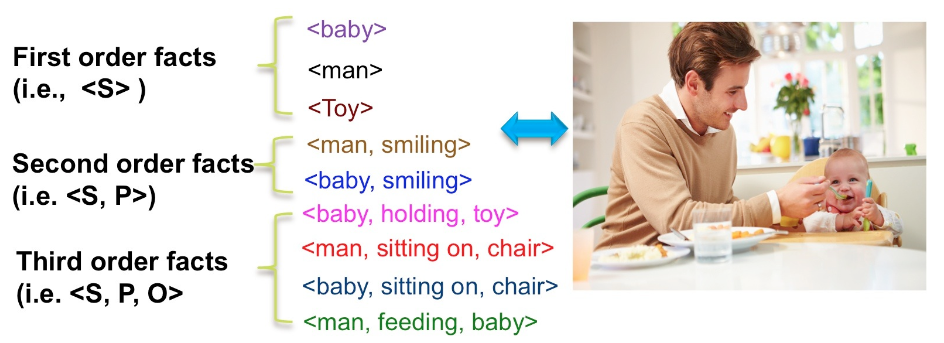 | Mohamed Elhoseiny, Scott Cohen, Walter Chang, Brian Price, Ahmed Elgammal, Sherlock: Scalable Fact Learning in Images, AAAI, 2017, acceptance rate (<25%). A new problem setting for never-ending structured fact learning. We propose a learning representation model that is able to learn facts of structured form of different types including first order facts (e.g., objects, scenes), second order facts (e.g., actions and attributes), and third order facts (e.g., interactions and two-way positional facts). We also propose an automatic method to collect structured facts from datasets of images with unstructured captions.
| AAAI2017 Conference paper github link : https://github.com/mhelhoseiny/sherlock Language & Vision Area https://sites.google.com/site/mhelhoseiny/sherlock_project
pdf Training pipeline and code https://www.dropbox.com/s/1ljv6qqxvyotemq/sherlock.zip
Download Benchmarked Developed in this work 1) 6DS benchmark (2.4 GB) (28,000 images, 186 unique facts) wit the training and testing splits Fact Recognition Top 1 Accuracy (our method): 69.63% Fact Recognition MAP/MAP100 (our method): 34.86%/ 50.68% 2) LSC (Large Scale benchmark) (814K images, 202K unique facts) part 1,LSC_dataset.tar.gz.aa (11.72 GB): part 2, LSC_dataset.tar.gz.ab (8.73 GB): After download, run cat LSC_dataset.tar.gz.* > LSC_dataset.tar.gz Then extract LSC_dataset.tar.gz with the training and testing splits
Fact Recognition Top 1 Accuracy (our method): 16.39% Fact Recognition MAP/MAP100 (our method): 1.0%
Models 1) Model 2 trained on LSC benchmark caffemodel deploy_prototxt
2) Code by Rohit Shinde to extract sherlock 900 dimensional features (300 S, 300 P, 300 O).
Feel free to contact for any questions. will add more materials later
| | Mohamed Elhoseiny, Ahmed Elgammal, Babak Saleh, Write a Classifier: Predicting Visual Classifiers from Unstructured Text , TPAMI, 2017
| Journal paper pdf Language & Vision Area (coming soon) | 2016 | | | | Mohamed Elhoseiny,"Language Guided Visual Perception", PhD Thesis in Computer Science, Rutgers University, October, 2016. | | | Mohamed Elhoseiny, Tarek El-Gaaly, Amr Bakry, Ahmed Elgammal, A Comparative Analysis and Study of Multiview Convolutional Neural Network Models for Joint Object Categorization and Pose Estimation, ICML,2016, oral presentation. | Conference paper Deep Learning Models  Download Analyzed CNN Models
|
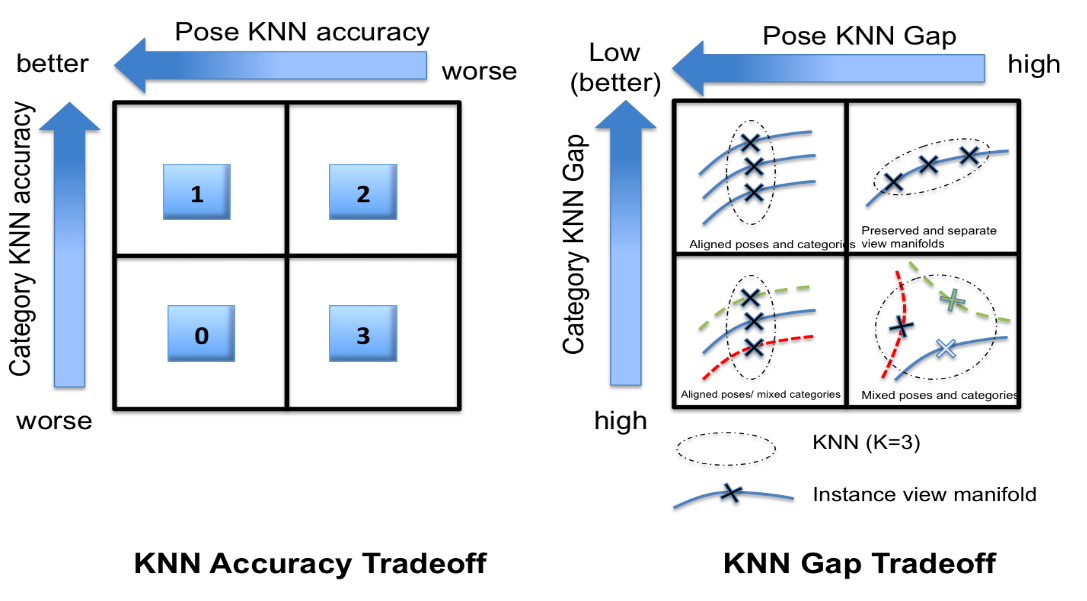 | Amr Bakry*, Mohamed Elhoseiny*, Tarek El-Gaaly*, Ahmed Elgammal, Digging Deep into the layers of CNNs: In Search of How CNNs Achieve View Invariance, ICLR, 2016, (overall acceptance rate is 24%) An study of how the CNN layers untangle the manifolderl of object and pose in the problem of joint object and pose recognition.
| Conference paper Deep Learning (Understanding Learning Representations for View Invariance) Understanding Deep Learning for Joint Pose and Object Recognition  | | Mohamed Elhoseiny, Jingen Liu,Hui Cheng, Harpreet Sawhney, Ahmed Elgammal Zero Shot Event Detection by Multimodal Distributional Semantic Embedding of Videos , AAAI, 2016 (acceptance rate is 549/2132=25.6%), oral presentation.
Detection of unseen events in videos by distributional semantic embedding of all video modalities into the same space. Our method can beat the state of the art with only the event title rather than a big text description used in prior work. It is also 26X faster. Evaluated on the large TRECVID MED dataset. | Conference paper Language & Vision Language & Video
paper  supplementary  Project page | | Mohamed Elhoseiny, Tarek El-Gaaly, Amr Bakry, Ahmed Elgammal, Convolutional Models for Joint Object Categorization and Pose Estimation, Arxiv, 2016 (non-archived presentation at ICLR Workshop Track presentation). Different convolutional neural network models are studied/proposed in this work to solve the problem of jointly predicting the class and the pose of an object given an image. 4 CNN models are proposed and evaluated on RGBD and Pascal3D datasets.
| Joint Pose and Object Recognition 
| | Han Zhang, Tao Xu, Mohamed Elhoseiny, Xiaolei Huang, Shaoting Zhang, Ahmed Elgammal, Dimitris Metaxas, SPDA-CNN: Unifying Semantic Part Detection and Abstraction for Fine-grained Recognition, CVPR, 2016 A part-based CNN method to jointly detect and recognize birds with small parts (7 parts). It also learn part based learning representation for each part that could be useful for other applications.
| Conference paper Deep Learning Detection of Bird parts.
 *Novel: part based learning representation
| | Mohamed Elhoseiny, Scott Cohen, Walter Chang, Brian Price, Ahmed Elgammal, Automatic Annotation of Structured Facts in Images, ACL Proceedings of the Vision&Language Workshop, 2016 (long paper, oral)
Motivated by the application of fact-level image understanding, we present an automatic method for data collection of structured visual facts from images with captions. Example structured facts include attributed objects (e.g., <flower, red>), actions (e.g., <baby, smile>), interactions (e.g., <man, walking, dog>), and positional information (e.g., <vase, on, table>). The collected annotations are in the form of fact-image pairs (e.g.,<man, walking, dog> and an image region containing this fact). With a language approach, the proposed method is able to collect hundreds of thousands of visual fact annotations with accuracy of 83% according to human judgment. Our method automatically collected more than 380,000 visual fact annotations and more than 110,000 unique visual facts from images with captions and localized them in images in less than one day of processing time on standard CPU platforms.
mages | ACL VL16 paper Language & Vision
 project link
Download Data collected by the Method from MSCOCO and Flickr30K datasets Download Link | 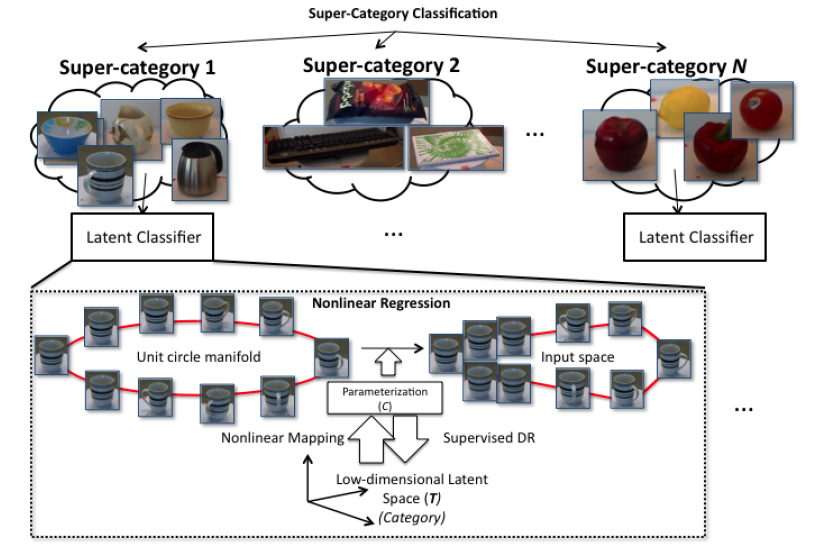 | Amr Bakry, Tarek El-Gaaly, Mohamed Elhoseiny, Ahmed Elgammal Joint Object Recognition and Pose Estimation using a Nonlinear View-invariant Latent Generative Model , WACV, 2016, Algorithms Track, acceptance rate 30% for algorithms track.
Recognition of class and pose from an image by a view-invariant latent Generative model.
| Conference paper Joint Pose and Object Recognition | 2015 | | |
| Mohamed Elhoseiny, and Ahmed Elgammal Overlapping Domain Cover for Scalable and Accurate Kernel Regression Machines, BMVC, 2015, oral presentation (acceptance rate 7%).
A novel method to that represent the data by an overlapping cover and enables kernel methods to be scalable to hundreds or thousands of images using the Largest Human3.6M dataset for pose estimation. | Conference paper Machine Learning Scalable Kernel Methods paper  extended abstract  supplementary  oral presentation
Project page
|
|
Sheng Huang, Mohamed Elhoseiny, and Ahmed Elgammal, Dan Yang Learning Hypergraph-regularized Attribute Predictors, CVPR, 2015, acceptance ratio 28.4%
A hyper-graph model for attribute based classification including attribute prediction, zero and n-shot learning | Conference paper Attribute Prediction and Zero Shot Learning
, code and Project page
| | Mohamed Elhoseiny and Ahmed Elgammal, Generalized Twin Gaussian Processes using Sharma-Mittal Divergence, ( ECML-PKDD in the Machine Learning Journal Track), 2015 . The paper will be orally presented at ECML-PKDD 2015 (journal track acceptance rate is <10%).
Using Sharma-Mittal divegranece, a relative entropy measure brought from Physics, to perform structured regression. Sharma Mittal divergence is generalized over several existing measures including KL, Tsallis, Renyi. Evaluated on two toy examples, three datasets (USPS, Poser, Human Eva). | Conference paper and Journal paper Machine Learning paper  oral presentation
project link
| 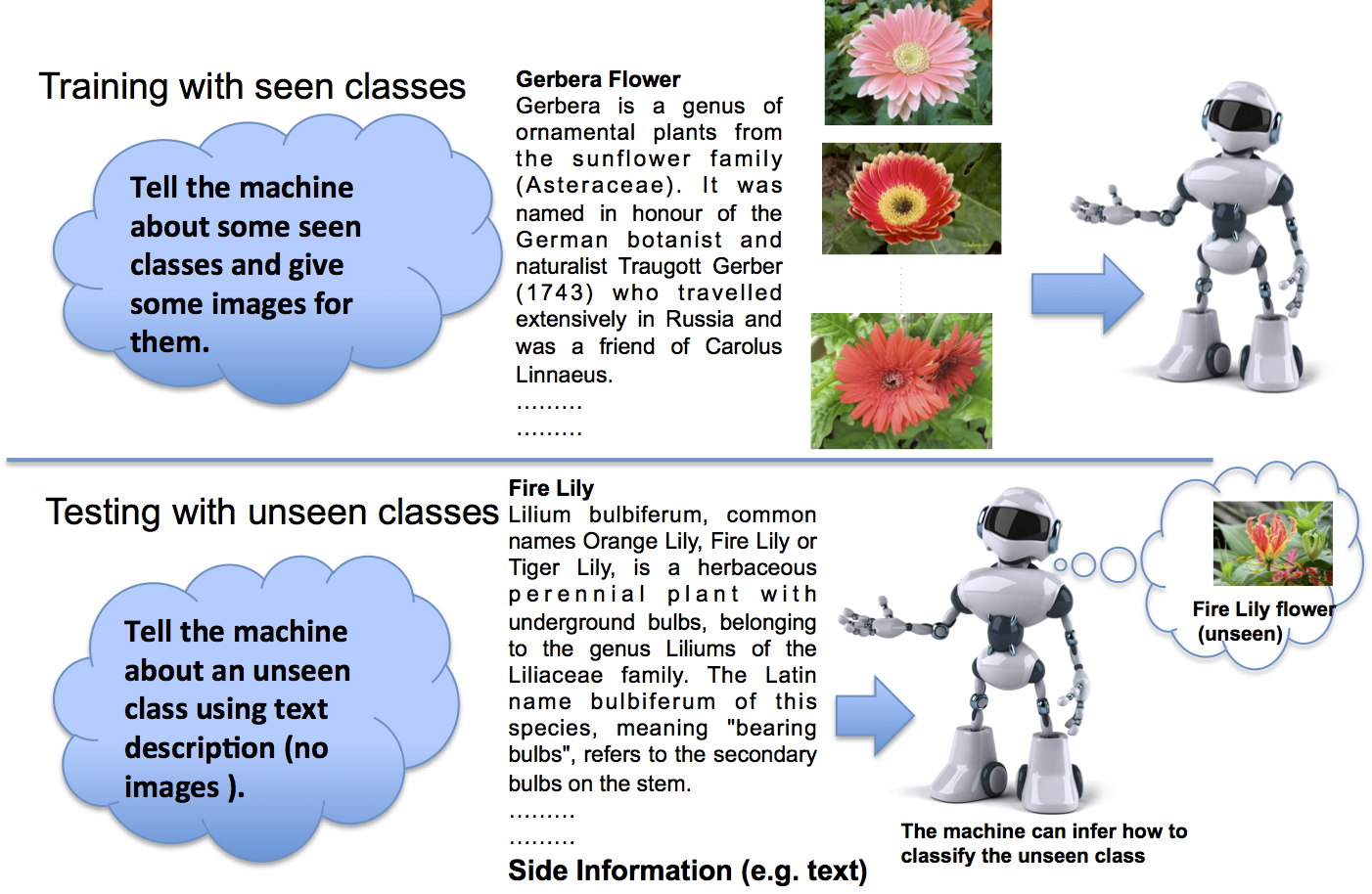 | Mohamed Elhoseiny, Babak Saleh ,Ahmed Elgammal, Tell and Predict: Kernel Classifier Prediction for Unseen Visual Classes from Unstructured Text Descriptions, Arxiv, 2015 This work was covered in a presentation in the CVPR15 Workshop on Language and Vision http://languageandvision.com/
Classification of unseen visual classes from their textual description for fine-grained categories | Workshop paper Language & Vision kernel version 
journal version of both linear(ICCV13) and kernel version presented in CVPRW15 and EMNLPW15 Project page and code | | Mohamed Elhoseiny, Ahmed Elgammal, Visual Classifier Prediction by Distributional Semantic Embedding of TextDescriptions, EMNLP Workshop on Language and Vision, 2015
The presentation focus on a newly proposed kernel between text descriptions which is part of the work detailed in , which could be useful for other applications
| Workshop paper Language & Vision presentation abstract 
| | Mohamed Elhoseiny, Sheng Huang, and Ahmed Elgammal Weather Classification with deep Convolutional Neural Networks, ICIP (Oral), 2015
Using and analyzing CNN layers for the weather classification. Our work achieves 82.2%normalized classification accuracy instead of 53.1% for the state of the art (i.e., 54.8% relative improvement) | Conference paper Weather Classification CNN paper  oral presentation Downloads (1) caffe CNN models (1GB) (2) Caffe trainvaltest prototxt (for training and loading the caffe models) project link
| 2014 | | | | Sheng Huang, Mohamed Elhoseiny, Ahmed Elgammal, Dan Yang, Improving Non-Negative Matrix Factorization via Ranking Its Bases, International Conference in Image Processing (ICIP), 2014 (Acceptance Ratio: 44.0%)
A Non-negative matrix factorization method by de-correlating bases and reranking them. | Conference paper Machine Learning
 | 2013 | | | |
Mohamed Elhoseiny, Babak Saleh, Ahmed Elgammal, Write a Classifier: Zero Shot Learning Using Purely Textual Descriptions, International Conference on Computer Vision (ICCV), 2013 (Acceptance Ratio: 27.8%)
The first work on classification of unseen visual classes from their textual description for fine-grained categories. We propose the first dataset in this problem; see the project link. | Conference paper Language & Vision
 project link
| | Mohamed Elhoseiny, Babak Saleh, Ahmed Elgammal, Heterogeneous Domain Adaptation: Learning Visual Classifiers from Textual Description Visual Domain Adaptation Workshop in Conjunction with ICCV 2013.
Additional experiments for our "Write a Classifier" work
| Workshop paper Language & Vision
 project link
| | Mohamed Elhoseiny, Bing Song, Jeremi Sudol, David McKinnon, Low-Bitrate Benefits of JPEG Compression on SIFT Recognition International Conference in Image Processing (ICIP), 2013 (Acceptance Ratio: 44%)
A study of the effect of JPEG compression on image matching performance. The main conclusion is that images with high compression ratio can be still recognized with SIFT. This enables low-bandwidth communication. | Conference paper Signal Processing
 | | Mohamed ELhoseiny, Amr Bakry, Ahmed ELgammal, MultiClass Object Classification in Video Surveillance Systems - Experimental Study, Oral Socially Intelligent Surveillance and Monitoring Workshop in Conjunction with IEEE International Conference on Computer Vision and Pattern Recognition (CVPR) 2013.
A study of different learning representation and latent variable analysis method for object classification in videos. | Workshop paper Recognition
 Download Data https://www.dropbox.com/sh/1tud115qsd1nh6n/AACx3YeDG-SeN7orb69hUUqia?dl=0
| Before 2013 |
Marwa Abdelmonem, Mohamed H.Elhoseiny, Asmaa Ali, Karim Emara, Habiba Abdel Hafez, Asmaa Gamal, Dynamic Optical Braille Recognition (OBR) System, International Conference on Image Processing and Computer Vision (IPCV), 2009
Optical Braille Recognition system (without fixed size constraint) | Conference paper Recognition
 | | | | | | |
II) Natural Language Processing and Multimedia Work (Automatic MindMap Generation from text) project link 2015 | Mohamed ELhoseiny and Ahmed Elgammal, Text to Multi-level MindMaps: A Novel Method for Hierarchical Visual Abstraction of Natural Language Text, International Journal of Multimedia Tools and Application (MTAP), 2015
An extended journal version of our "English2MindMap" work, much more details included . The work serves as new way to hierarchically visualize Text description by pictures in multiple levels. | Journal paper Text to MindMap as a Hierarchical Visual Abstraction
 project link | 2013 |
Mohamed H.ElHoseiny, Ahmed Elgammal, English2MindMap: Automated system for Mind Map generation from Text, Oral, International Symposium of Multimedia (ISM), 2012 Also presented in the NYC Multimedia and Vision Meeting, 2013
The first work on generating Multi-level MindMap from text. | Conference paper Text to MindMap
 project link | before 2013 | Asmaa Hamdy, Mohamed H. ElHoseiny, Radwa Elsahn, Eslam Kamal, Mind Map Automation (MMA) System, International Conference on Semantic Web and Web Services (SWWS), 2009.
A demo for an early work to automate MindMaps from text description. | Conference paper Demo Project
 project link |
III) GPU Work Technical Reports 2013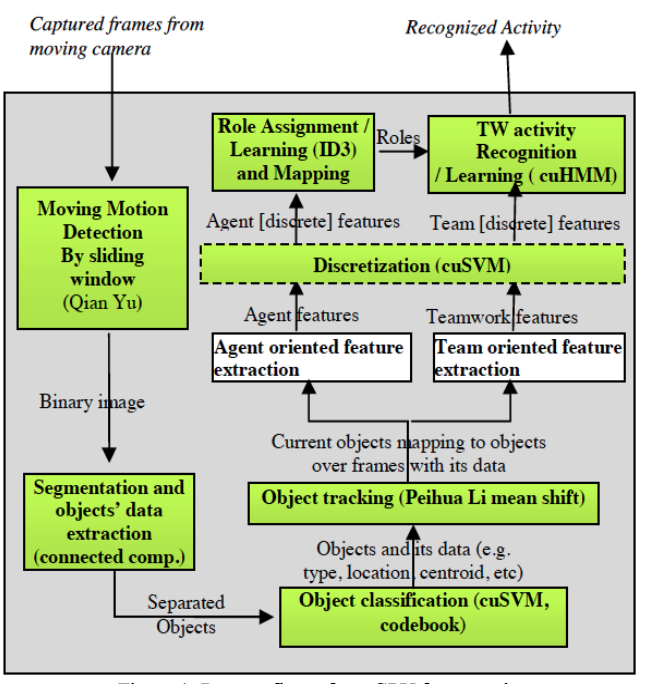 |
Mohamed H. ElHoseiny, H.M.Faheem, Eman Shaaban, T.M. Nazmy, GPU framework for Teamwork Action Recognition arXiv, 2013
A GPU framework of activity recognition that works 20X faster without GPU for this task | Technical Report
 | Before 2013 | Mohamed H. ElHoseiny, High Performance Activity Monitoring for Scenes Including MultiAgents (Humans and Objects) Faculty of Computer and Information Sciences, Ain Shams University, 2010. | Thesis
|
Bachelor Project | Mohammad Saber AbdelFattah*, Mohammad H. Elhoseiny*, Hatem AbdelGhany Mahmoud*, Mohammad El-Sayed Fathy*, Omar Mohammad Othman*, Compiler Construction WorkBench Bachelor thesis, Faculty of Computer and Information Sciences, Ain Shams University, 2006. *Equal Contribution
Bachelor project where we built several tools for compiler generation. |
 |
|
Mohammad Elhoseiny, Dec 17, 2010, 4:19 AM v.2 Mohammad Elhoseiny, Dec 15, 2012, 11:20 AM v.2 Mohammad Elhoseiny, Dec 17, 2010, 4:19 AM v.2 |